Why I’m (still) hyped about the Algorithmic Accountability Act
Update! The Algorithmic Accountability Act was re-introduced September 21, 2023 by Senators Wyden and Booker and Representative Clarke along with 14 other co-sponsors.
I spent the 2021 year serving as a technology advisor to Senator Ron Wyden (D-OR) and learning about being a staffer in Congress through the TechCongress Congressional Innovation Fellowship.
To apply to TechCongress, visit techcongress.io/apply. You can read about what motivated me to work in Congress and see my answers to the 2021 cohort application as a reference.
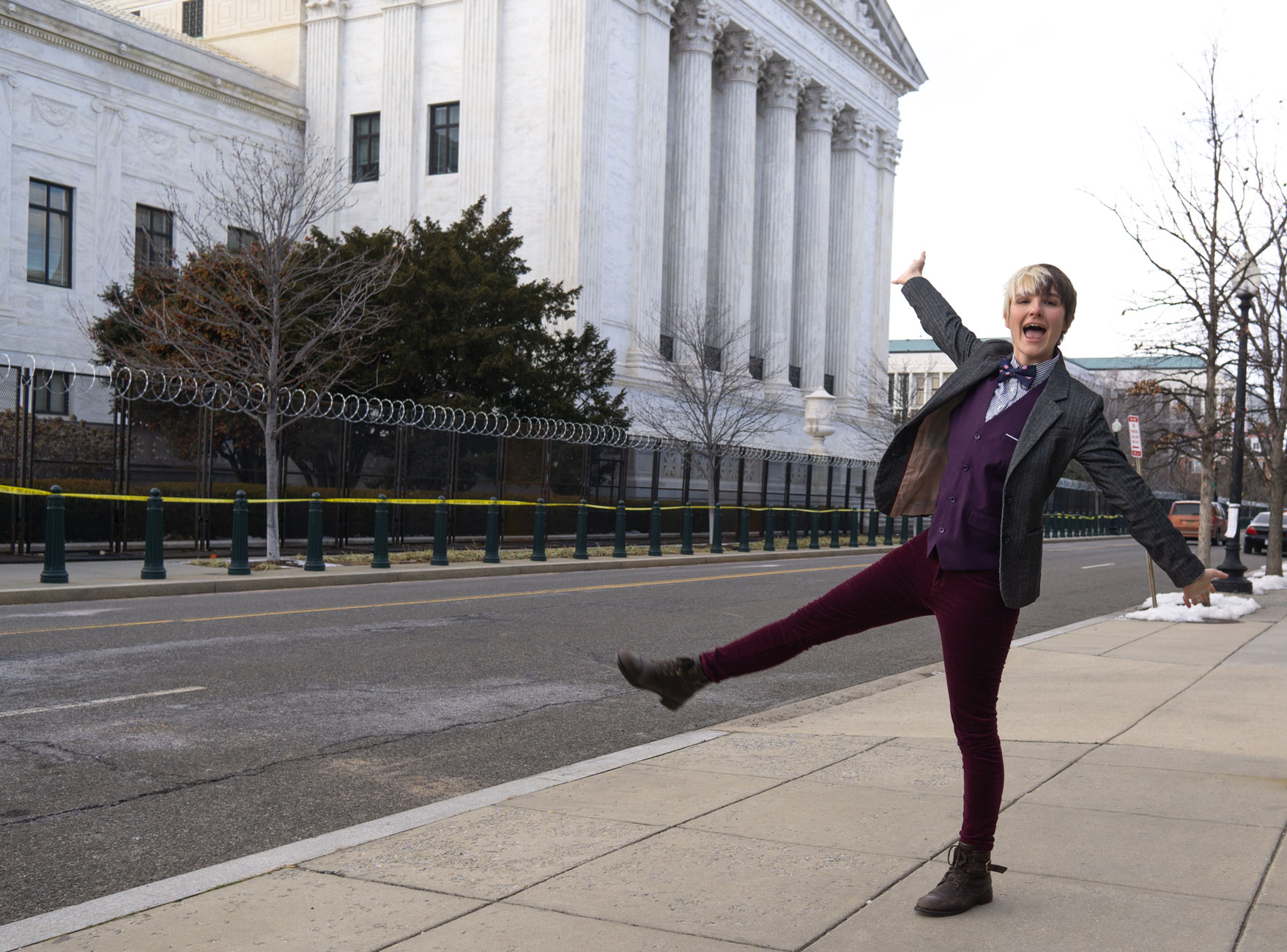
I decided to share what I think makes this bill really interesting and exciting and highlight some stuff I think makes it an important piece of legislation not just for AI governance but for how we think about technology policy more broadly. I also wanted to explain some of the thinking behind the bill in case it might inspire others or challenge people to re-examine how they approach policymakers to make change.
What follows is a cleaned up, edited, and expanded version of what was originally shared on Twitter (and later Mastodon).
Due to length, I’ve split it up into parts:
- Algorithmic Accountability from 10,000ft
- Why I’m (still) hyped about the Algorithmic Accountability Act of 2022 (that’s this!)
#1: Impact Assessment is an activity not an artifact
#2: Focus on decisions, not data types
#3: Three layers of disclosure - How to get into AI policy (coming soon)
Legislative text may not always have a reputation for being super exciting stuff. With all of the sections and subsections with references to other sections and subsections, it certainly can feel a little overwhelming to read. But Federal legislation can also be powerful, and I think that it can be really interesting if you understand how decisions about the particular words that make it up shape the ways that legislation may be interpreted.
For a recap of what the Algorithmic Accountability Act does, at a high level, and where it came from, check out Algorithmic Accountability from 10,000ft.
There’s so much we could get into, but for now I’ll be sharing three things that I am personally excited about in the approach taken in the Algorithmic Accountability Act of 2022.
LET’S DIG IN!
Thing I’m excited about #1: Impact Assessment is an activity not an artifact
One thing you may notice in reading the body of the bill is that it very rarely talks about impact assessment as a plural (“impact assessments”). This is because it treats impact assessment as a mass noun.
Didn’t think you were gonna get a grammar lesson, did ya?
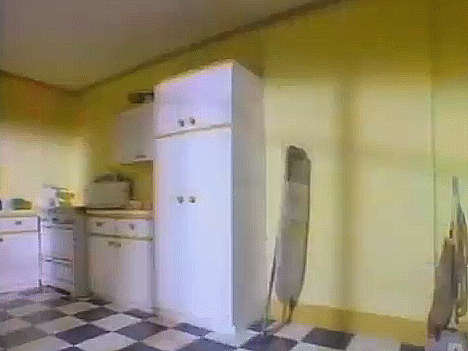
Mass nouns (also called noncount nouns) are words like diligence, management, information, feedback, hospitality, mail, poetry, software, training, legislation, or even… bacon! Mass nouns can be used to denote continuous rather than discrete entities. By describing “impact assessment” as a mass noun, this is an intentional shift away from thinking of assessments (plural) as discrete, individual units and toward something more continuous. We’re not talking about one-off events, but rather impact assessment as an ongoing activity.
One way I like to think of it is like documentation of code. When you’re developing software, there are sometimes discrete artifacts and resources that are produced (documents), but documentation is not just artifacts, it is a process of tracking changes, describing goals, and communicating functions. It is an ongoing activity throughout the lifecycle of software. Documentation may be updated every time a change is made or whenever something significant happens depending on the architecture and specifics of the process being documented.
Big shout outs to Jingying Yang, Timnit Gebru, Meg Mitchell, Hanna Wallach, Jenn Wortman Vaughn, Deb Raji whose brilliant thinking on documentation for machine learning systems first exposed me to this concept through work like ABOUTML
This “activity not artifact” approach is important because we know that systems are dynamic and that both the technologies and the environments in which they operate are subject to change over time which will influence the impacts.
So that’s thing #1:
In the Algorithmic Accountability Act, impact assessment is a process, a set of actions, an ongoing activity that is integral to deploying automated decision systems to make critical decisions.
Thing I’m excited about #2: Focus on decisions, not data types
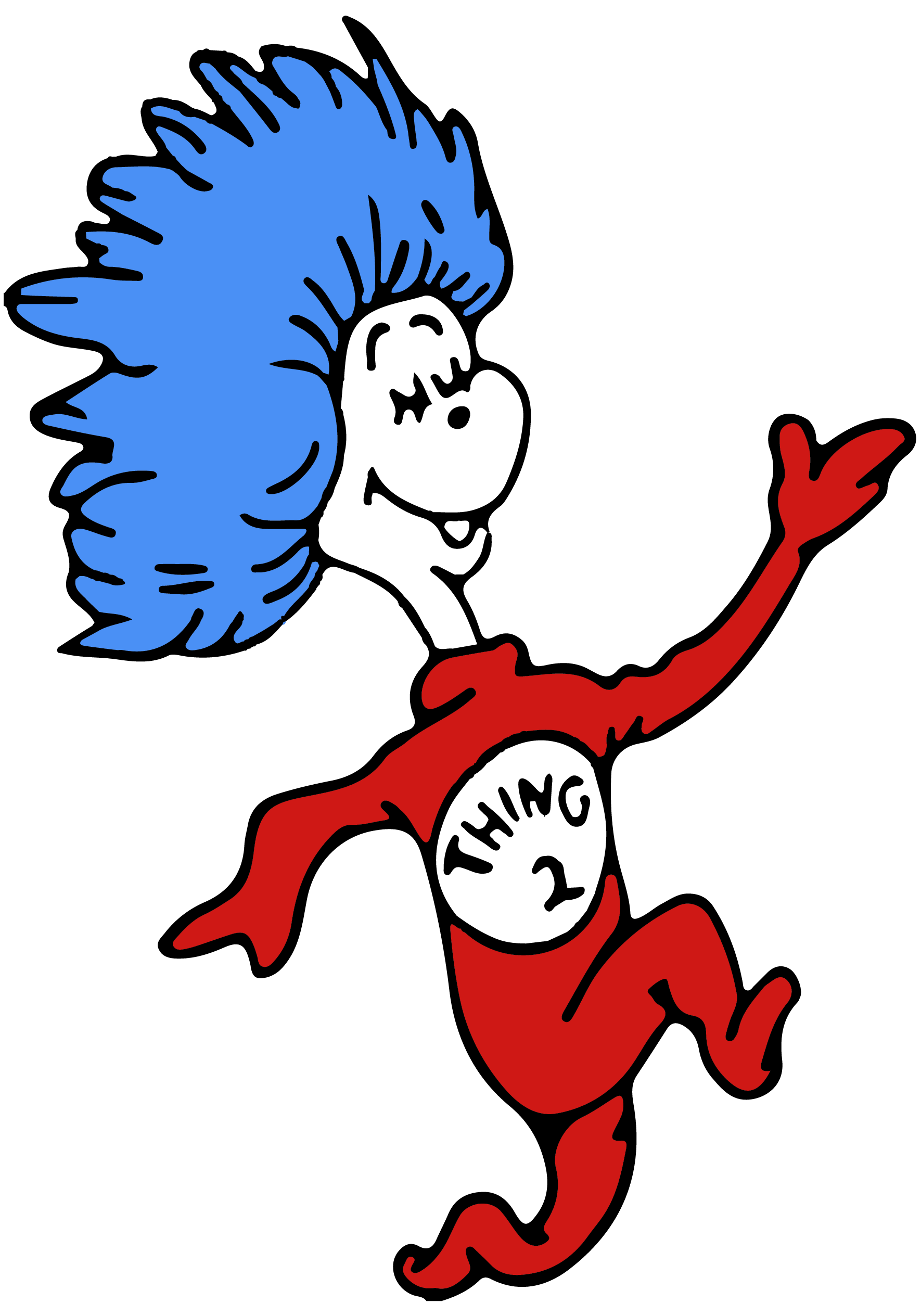
A pretty big shift from the 2019 version of the bill (and much of the legislation in this space) is the move away from the definition of “high-risk” systems to the frame of “critical decisions.”
Warning: this may seem a little pedantic at first, but when we’re talking about stuff that may turn into law and be interpreted by the courts for decades to come, it pays to be specific!
The Algorithmic Accountability Act uses this framing of “critical decisions,” but a lot of legislation and regulation for AI and automated decision systems (ADS) uses “high-risk” systems. Before I get into why “critical decisions” might be preferable, let’s break down the criteria most often used to define “high-risk” systems and why—despite being appropriate in some other texts—they’re not appropriate for Algorithmic Accountability.
Here’s the thing about regulating “high-risk” systems, you kinda have to have an idea already about what is risky. The other legislation in this space tends to conceive of risk through three main criteria:
- number of people impacted
- sensitivity of data involved
- severity of impact
Let’s step through this list and talk about why each of these things can be either difficult to use in—or even possibly antithetical to the goals of–the Algorithmic Accountability Act and potentially other bills written to regulate these ADS technologies, as well.
a) Number of people impacted
One way to think about the risk of a system is related to the number of people who are impacted by that system. This makes a lot of sense for many applications, but for Algorithmic Accountability Act, using the number of people impacted to define risk just wouldn’t work.
Don’t get me wrong: I think this is an important thing to try to capture when thinking about what may make a system more or less risky, but for Algorithmic Accountability the issue is that until you have assessed a system, you may not know who all it impacts! You can’t define the type of system that is captured by a rule by something you don’t know until you actually apply the rule, so for Algorithmic Accountability, we had to take a different approach.
Sidebar: there’s a WHOLE other conversation to be had about how to define things like “number of users” that probably needs way more standardization because hoo boy! do people disagree on that. (Even a single company may have many different definitions & metrics for defining “number of users” across different teams!)
If you’re a staffer writing legislation trying to navigate this… Godspeed. For folks outside of Congress who want to make tech laws better, try writing some thought-out definitions for “users” in different contexts. It could really go a long way.
JUST SAYINGGG
b) Sensitivity of data involved
When you can’t define by the scale of impact (number of people), it can be tempting to focus on the types of permissible data instead. This is actually what the original 2019 version of the Algorithmic Accountability Act did.
There is so much established literature and law about sensitive data, personally identifying information, protected health information, and so forth! And for sure: there is a real, pressing need for data privacy legislation in the US. There are real harms that come from sensitive information being exposed or used irresponsibly, and the explosion of data collection about people makes this all the more urgent!
Privacy law is important and urgently needed, BUT privacy law and algorithmic accountability law are complementary causes, not substitutes for one-another. Not only does law and regulation for AI & ADS need to do different things, but sometimes the goals of privacy and algorithmic accountability are in tension!
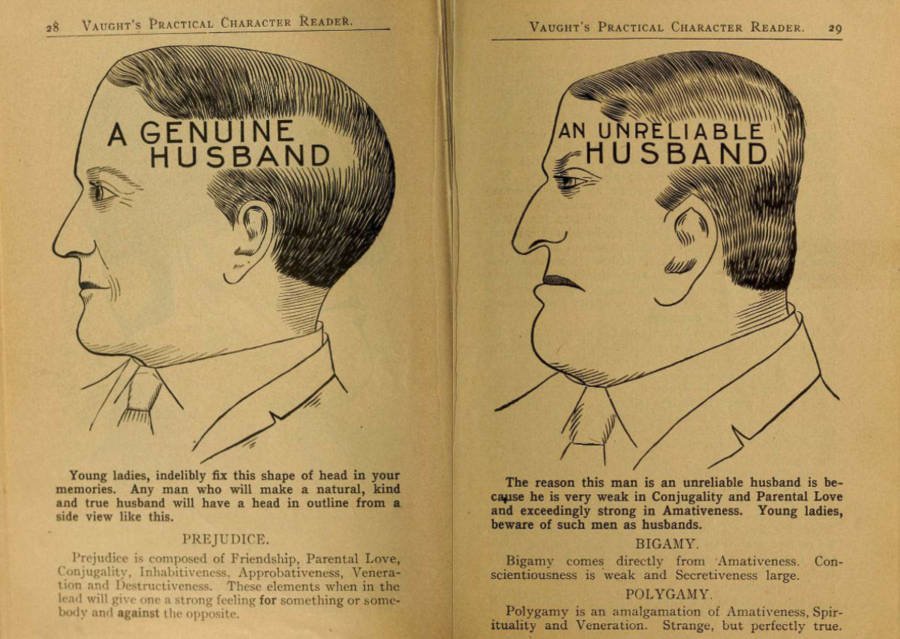
Regulating systems for making decisions based on the data INPUTS to those systems rather than their specific uses creates perverse incentives to use less ~sensitive~ data, even if that is the data actually most pertinent information to the situation. Making decisions using only benign information can still be dangerous. If a system is used for making critical decisions about a person’s healthcare, it probably SHOULD be using sensitive health information! Using more benign data (through proxies or straight up irrelevant info) may not only be unhelpful, it may actually harm people.
This is why focusing on “high-risk” systems as defined through data sensitivity is dangerous.
Finally: c) Severity of impacts
Severity of impacts is now probably one of the most common approaches for defining systems as “high-risk.” This also can make sense in some contexts, but wasn’t the right focus for the Algorithmic Accountability Act.
It’s worth noting that there are different types of bills (which turn into different types of laws). Because different bills have different goals, they may focus on addressing the same problems with different approaches. For example, some bills may try to address the negative impacts from ADS with a goal of providing recourse to people harmed. With that goal, measuring risk by severity of impact can be useful. This strategy may be applicable in cases where the impacts of ADS are well documented. It does depend, however, on knowledge about the impacts of using a system.
Not only do we not know what the impacts of many systems are yet, we actually don’t know all of the different sorts of systems out there. In Algorithmic Accountability, our goal is to UNCOVER the impacts in contexts where automated systems are used in order to identify harms (as well as positive impacts). Because this information isn’t known, focusing on this kind of “high-risk” system doesn’t work.
The alternative? Critical decisions
So we’ve talked through three of the main ways people classify ADS as “high-risk,” but you might be saying to yourself, “but none of those capture how the EU AI Act—one of the most significant pieces of legislation on algorithmic regulation out there—does it!” And you’d be right.
A second understanding of the severity-of-impacts framing is the potential for harm if a system doesn’t perform accurately or performs in a way that is biased or otherwise problematic. This is the approach that the EU AI Act takes, as I understand it. Its approach to “high-risk” systems is defined based upon the potential for a technological system to be the source of harm. This is certainly one of the ways that a system could be “high-risk,” but in this section, I hope to communicate why I think focusing on “high risk” systems is actually incomplete, and why the “critical decision” framework matters. It’s a little subtle, but I think it’s really important!
As we covered above, the Algorithmic Accountability Act of 2022, doesn’t define its automated systems of interest by 1) number of people impacted, 2) sensitivity of data used, or even 3) severity of impact. In fact, if you look closely, you might notice something peculiar. The Algorithmic Accountability Act of 2022 isn’t really about algorithms.
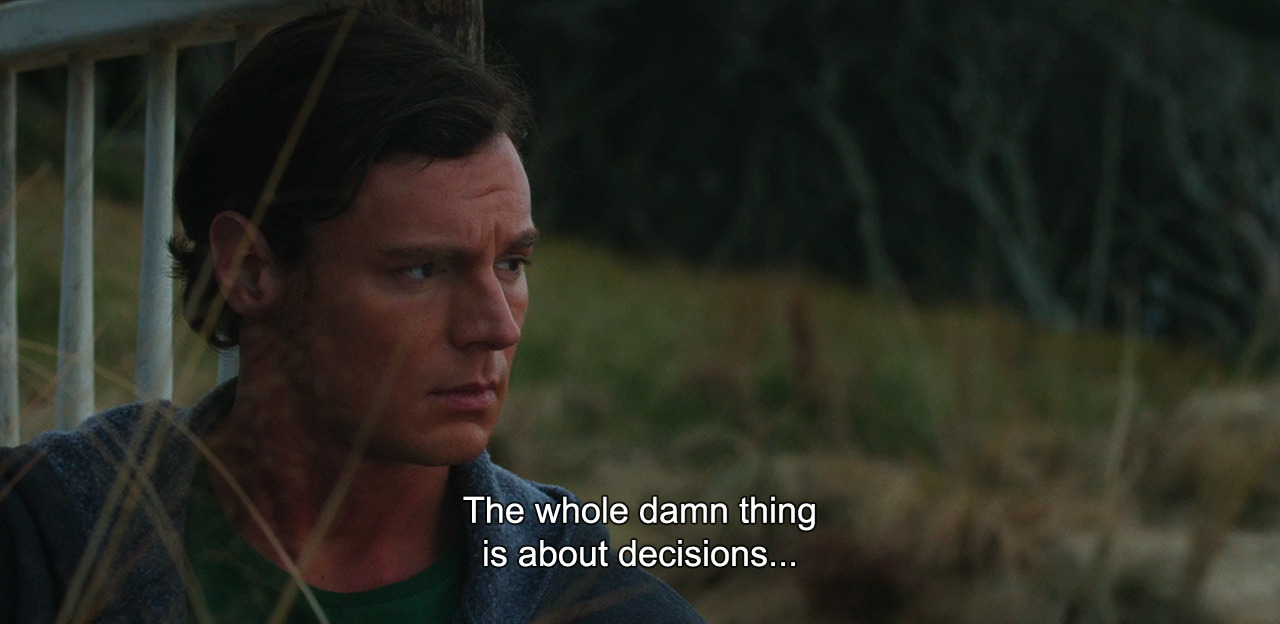
Instead of focusing on particular systems, most of Algorithmic Accountability is written about “augmented critical decision processes.” So let’s unpack that!
As described in Algorithmic Accountability from 10,000ft, an “augmented critical decision process” is a process where an “automated decision system” is used to make a “critical decision.” Said another way: an augmented critical decision process (or ACDP) is a process where computational systems are used, the results of which serve as a basis for a decision or judgment about the cost, terms, or availability of a bunch of critical stuff in people’s lives like education, employment, healthcare, and housing.
These ACDPs are referred to throughout the bill (62 times, in fact!) because the Algorithmic Accountability Act recognizes that harms caused when employing ADS to make critical decisions may not only come from the ADS. Instead, Algorithmic Accountability recognizes that automation has the capacity to scale up and speed up existing harmful processes, often while obfuscating the actual decision makers, making accountability more challenging.
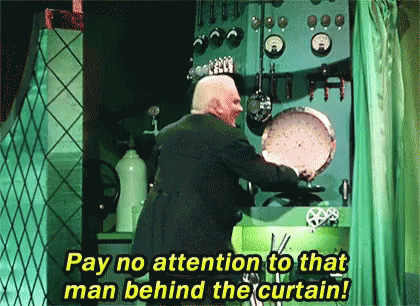
Therefore, Algorithmic Accountability doesn’t just require assessing the impacts of automated decision systems, but rather it requires that we assess the impact of the whole ACDP, the whole critical decision process that is being automated, to document how these decision processes work and the role that ADS plays. By doing this, we not only hope to better understand these processes but, hopefully, can identify and mitigate any harms uncovered along the way.
So, even though the categories used in the EU AI Act and Algorithmic Accountability Act of 2022 may appear similar, the targets of assessment are actually subtly, but importantly different.
Another thing that the ACDP framing accomplishes is that it also narrows the scope somewhat. This is important for potentially boring government-y reasons because, after all, this is an FTC bill.
You may notice that the list of things that make up these “critical decisions” does exclude some things that many people might expect to see in AI/ADS laws (like some of what’s in the EU AI Act).
This is related to that boring government jurisdictional stuff. Since the FTC is about consumer protection it doesn’t cover government use like the criminal legal system, immigration, or government benefits administration.
So that’s thing #2:
The Algorithmic Accountability Act isn’t about governing types of data or types of impact, but rather assessing the impacts of making certain types of decisions with the help of automated technologies.
So that brings us (finally!) to…
Thing I’m excited about #3: Three layers of disclosure
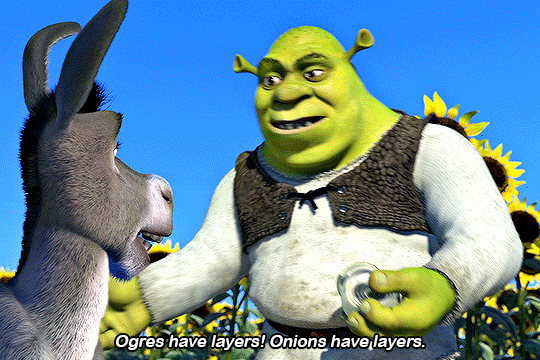
One big critique of the 2019 version of the Algorithmic Accountability Act was that it did not include reporting on the impact assessment behaviors of covered entities (aka companies). Reporting is an important element to accountability because it offers a level of transparency into processes and it introduces procedural checks to ensure that impact assessment is, indeed, taking place. (Impact assessment is different from some other approaches like audits, licensing, etc, but these things are not mutually exclusive.)
I imagine this is one of the SPICIER elements of the bill, so let’s discuss!
The new version of Algorithmic Accountability has new disclosure requirements in three layers:
- internal assessment of impact within companies (an ongoing activity, remember?)
- summary reports (a particular artifact that comes out of that activity) submitted to the FTC
- information shared by the FTC to the public in the form of:
- aggregated anonymized trend reports that the FTC produces
- a searchable repository with key (identifying) info about the reports received
Before we get into why this is a Thing I’m Excited About, let’s first talk about what many people want this bill to do (which is doesn’t do), and then I’ll tell you why I think that THIS is actually even better!
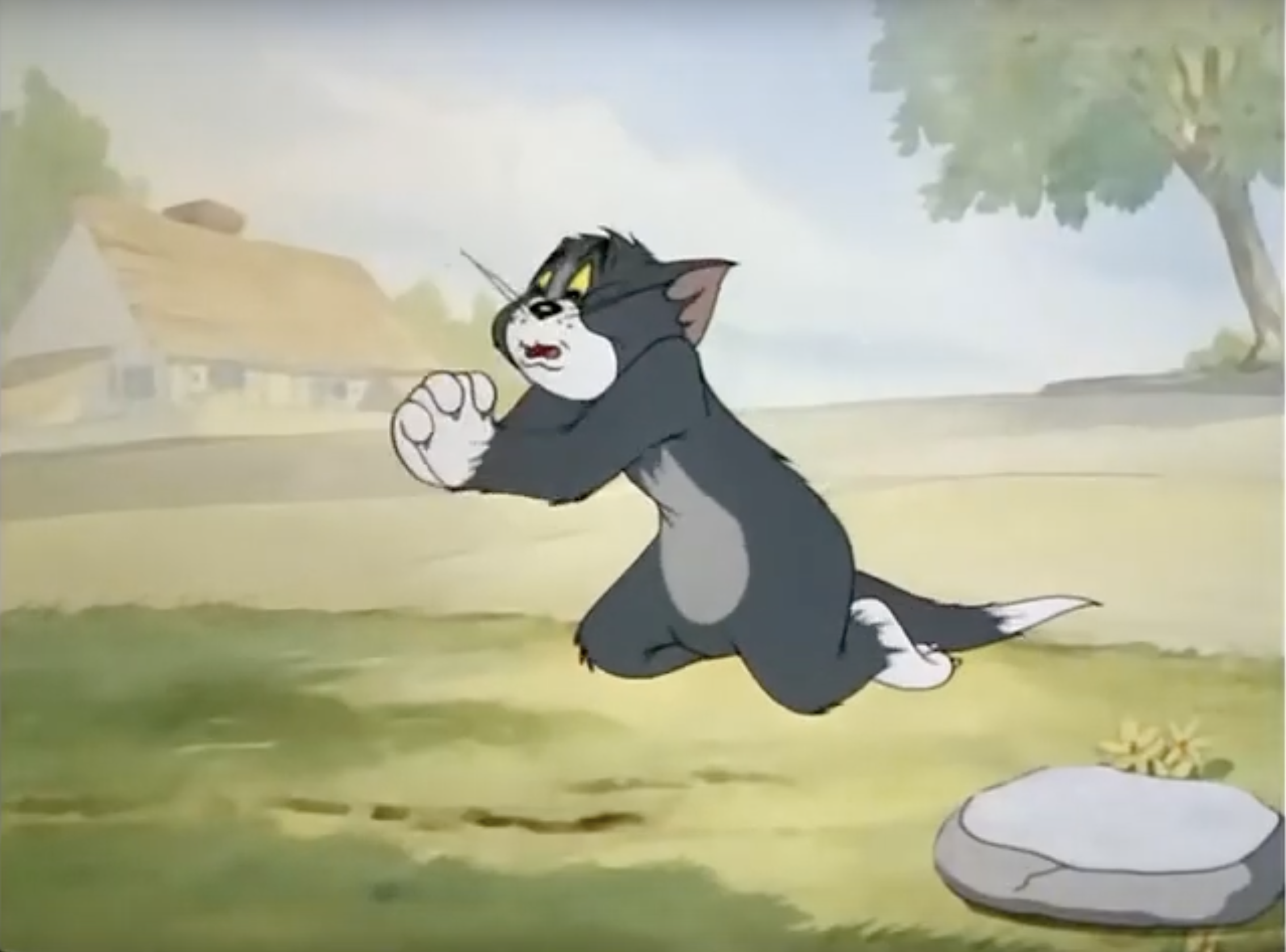
(warning: caricature incoming)
A lot of people want Algorithmic Accountability to be about catching bad actors red-handed. They want to expose and name-and-shame those who are allowing their automated systems to amplify and exacerbate harms to people. This is righteous, and I empathize. I also want there to be justice for those harmed, and I also want there to be real consequences for causing harm that willful and feigned ignorance do not excuse.
I do believe that this is a step in that direction, but this bill focuses on something slightly different: Algorithmic Accountability is less about helping the FTC catch wrongdoers, (although there is room for that, and I’ll explain more) and it’s more about making it easier and more clear on how to do the right thing.
One of the great challenges in addressing the impacts of automated decision systems is that there is not (yet!) an agreed upon definition of what “good” (or even “good enough”) looks like. We lack standards for evaluating decision systems’ performance, fairness, etc. Worse still, it’s all super contextual to the type of decision being made, the types of information/data available, etc. These standards may one day exist! But they don’t yet. Algorithmic Accountability is about getting us there.
And part of getting there, I believe, is facilitated through the three tiers of disclosure and reporting.
Layer 1: Internal assessment of impact within companies
This comes back to what we talked about in Exciting Thing #1: impact assessment is a process, an ongoing activity, an integral part of responsible automated decision product development and deployment. The Algorithmic Accountability Act of 2022 requires all companies that meet certain criteria to do this and keep records internally of their processes.
Layer 2: Private submission of summary reports to the FTC
Now here comes the potentially ~spicy~ bit!
The bill requires companies to submit documentation substantiating their impact assessment activities to the FTC. (To see what’s required, peep Section 5.) This submission is done PRIVATELY, meaning that it’s between the government and the one submitting one company, here.
This documentation is required to be submitted before a company deploys—as in sells/licenses/etc OR uses, themselves—any NEW automated decision system for the purpose of making critical decisions. It is also required annually for any existing system as long as it is deployed. This reflects the continuous nature (the mass noun!) of impact assessment we talked about earlier. It is an ongoing activity, but these summary docs are snapshots of that activity in action.
Many folks may feel these reports should be made entirely public. I get where that’s coming from, but here’s why I think this private reporting to the FTC is actually a kinda clever way to go about it…
- Because we lack standards, it is premature to codify specific blanket requirements for which specific metrics for evaluating performance, for instance, all companies should use. As such, companies will likely choose whichever ones make them look “best” meaning people won’t put out damning info.
To be clear: this kinda "metric-hacking” is to be expected, and whether the reports are private or not, companies (out of fear of accountability or at least judgment) will probably assess impacts and use the metrics that they think will likely reduce the likelihood that they get called out. Such is the nature of humans (especially within a punitive framework)!
- (Okay, now here’s the fun part!) Because these reports are submitted privately to the FTC, companies are now in a position of information asymmetry. They do not know what OTHER companies are saying they did or how they performed on THEIR metrics. They may try to do the bare minimum, but they don’t actually know what the bare minimum is!
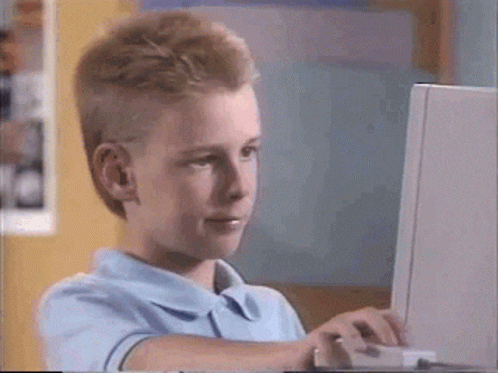
Gotta love it when collective action problems work on our side! 😜
The FTC (plus some other agencies), however, get to see across the collection. And this is super useful! Not because companies are going to “tell on themselves,” (they will try incredibly hard to not do that) but because there are really interesting lessons to be learned from how different companies fulfill these requirements. There is as much to be learned from what particular companies do say in their reports as what they don’t. The information asymmetry makes this more JUICY!
See, right now there’s a dynamic where any company (or more like employee) that tries to really interrogate the impacts of these automated decision technologies gets called out for it. Inevitably, doing honest impact assessment will turn up some… room for improvement. But recognizing where things are going wrong is the first step in the process of doing something about it.
At the moment, though, asking the tough questions and being open about challenges makes one a target. It’s a “tall poppy” situation. It’s better to not know, to not try, than to find out the truth. The companies that do the least don’t make headlines. The automated decision systems that no one knows about don’t feature in hashtags. The current culture around responsible tech rewards keeping your head down, not asking questions, and staying obscure. It often punishes those that try to ask, to measure, to identify and prevent harm.
This private reporting dynamic shifts that calculus.
With the Layer 2 reporting constraints, companies aren’t telling on themselves so much as they’re telling on each other. By doing more thorough assessment compared to industry peers, companies make those OTHER companies look worse rather than themselves. This competition could even reduce collusion pressures. With Algorithmic Accountability, there is an opportunity for a race to the top that doesn’t exist in the current equilibrium.
Maybe you think that this is all just “going through the motions,” and this reporting is just a song-and-dance that won’t make any REAL difference. I guess it’s possible, but even “going through the motions” can save lives. Honestly, there’s so much BASIC stuff out there that hurts people that could be avoided if people were even just a little bit conscious of it when designing, developing, and using powerful automation tools. Even the much-maligned “checklist” for impact assessment can be a powerful tool if it provides air cover for well-intentioned employees of companies to work in the public interest.
Maybe you say “but still, there are things that The Public really does deserve to know!” And it’s true. Some things are really essential. Like knowing what decisions about you are being automated or knowing if there is a way to contest or correct one of these decisions.
And so…
Layer 3: Information shared by the FTC to the public
There are two complementary components to the reporting Layer 3:
- Aggregate anonymous trend reports
- A searchable repository.
Hop over to Section 6 of the Algorithmic Accountability Act to see detailed information about what information will be made public about companies’ use of automated decision systems to make critical decisions about people’s healthcare, education, and more!
The Algorithmic Accountability Act is a consumer protection bill (where consumer is defined as… any person. Turns out there’s no official FTC definition of consumer! 😜) Part of that consumer protection comes from making key information available to the public in a place where individuals—and also awesome consumer-protection and advocacy organizations—can access it.
This 3rd tier of disclosure consists of two different flavors of information. One is an information-rich, qualitative report of the findings and learnings aggregated from the multitude of individual reports. This is where the FTC can highlight differences and patterns.
Personally, I’m really interested to learn about things like… do different critical decisions (health vs employment) gravitate toward different metrics for evaluating performance? What types of stakeholders are being consulted with? How?
The second half of Layer 3 is the public repository. This has more limited information, but contains a record for every critical decision that has been reported and contains that key information we alluded to earlier. The repository must “allow users to sort and search the repository by multiple characteristics (such as by covered entity, date reported, or category of critical decision) simultaneously,” ensuring that it can be a powerful resource for both consumers and advocates as well as researchers.
Together, these three layers of information disclosure can provide an opportunity to 1) catch issues early where companies can still fix, 2) motivate a greater “race to the top” on both how automated decision systems are used and on impact assessment, itself, & 3) provide the public with essential information for making better-informed choices and for holding companies accountable.
And that’s thing #3:
The Algorithmic Accountability Act uses three different layers of information disclosure to maximize the impact of assessment.
There you have it, folks!
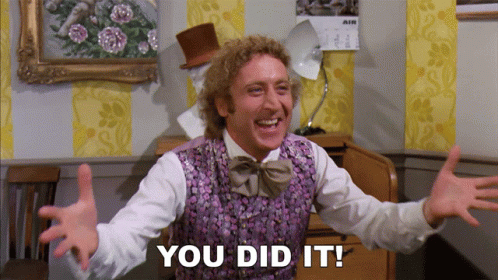
My big 3 reasons why I’m (still) hyped about the Algorithmic Accountability Act of 2022:
#1: Impact Assessment is an activity not an artifact
#2: Focus on decisions, not data types
#3: Three layers of disclosure
There’s a lot more to say, but I hope this breakdown helps illustrate some of the clever ways that writing robust legal definitions about tech and red-teaming regulatory requirements can potentially produce better legislation for tech policy issues!
Jump around:
- Algorithmic Accountability from 10,000ft
- Why I’m (still) hyped about the Algorithmic Accountability Act of 2022 (that’s this!)
#1: Impact Assessment is an activity not an artifact
#2: Focus on decisions, not data types
#3: Three layers of disclosure - How to get into AI policy (coming soon)